grand
乱数生成器
呼び出し手順
Y = grand(m, n, "bet", A, B) Y = grand(m, n, "bin", N, p) Y = grand(m, n, "nbn", N, p) Y = grand(m, n, "chi", Df) Y = grand(m, n, "nch", Df, Xnon) Y = grand(m, n, "exp", Av) Y = grand(m, n, "f", Dfn, Dfd) Y = grand(m, n, "nf", Dfn, Dfd, Xnon) Y = grand(m, n, "gam", shape, rate) Y = grand(m, n, "nor", Av, Sd) Y = grand(m, n, "geom", p) Y = grand(m, n, "poi", mu) Y = grand(m, n, "def") Y = grand(m, n, "unf", Low, High) Y = grand(m, n, "uin", Low, High) Y = grand(m, n, "lgi") Y = grand(m, n, o, …, PDFname, …) Y = grand(X, PDFname, …)
Y = grand(n, "mn", Mean, Cov) Y = grand(n, "markov", P, x0) Y = grand(n, "mul", nb, P) Y = grand(n, "prm", array)
S = grand("getgen") grand("setgen", gen) S = grand("getsd") grand("setsd", S) grand("setcgn", G) S = grand("getcgn") S = grand("phr2sd", phrase) grand("initgn", I) grand("setall", s1, s2, s3, s4) grand("advnst", K)
パラメータ
- m, n, o
integers, size of the wanted matrix / hypermatrix
Y
.- X
次元(つまり
m x n
) のみ使用される行列- PDFname
Name of the chosen probability Density Function:
"def", "unf", "uin", "bet", "gam","exp", "nor", "geo", "poi", "bin", "chi", "nbn", "nch", "f", "nf", "lgi"
.- Y
depending on the input, a matrix or hypermatrix, with random entries.
- S
処理の出力 (通常は文字列または実数の列ベクトル)
説明
警告: シードを指定しない場合,系列はあるセッションと別のセッションで 同一のままとなります.
rand関数を使用する各スクリプトの初めに, 以下を実行する必要があります:
grand(…)
generates random numbers from various probability distributions.
The syntaxes
Y = grand(m, n, "bet", A, B) Y = grand(m, n, "bin", N, p) Y = grand(m, n, "nbn", N, p) Y = grand(m, n, "chi", Df) Y = grand(m, n, "nch", Df, Xnon) Y = grand(m, n, "exp", Av) Y = grand(m, n, "f", Dfn, Dfd) Y = grand(m, n, "nf", Dfn, Dfd, Xnon) Y = grand(m, n, "gam", shape, rate) Y = grand(m, n, "nor", Av, Sd) Y = grand(m, n, "geom", p) Y = grand(m, n, "poi", mu) Y = grand(m, n, "def") Y = grand(m, n, "unf", Low, High) Y = grand(m, n, "uin", Low, High) Y = grand(m, n, "lgi")
m
x n
matrix with random entries.
The syntax
Y = grand(m, n, o, …, PDFname, …)
m
x n
x o
x... hypermatrix
with random entries.
Y = grand(X, PDFname, …)
size(X)
,
with random entries. X
is used only as template to size the result.
The syntaxes
Y = grand(n, "mn", Mean, Cov) Y = grand(n, "markov", P, x0) Y = grand(n, "mul", nb, P) Y = grand(n, "prm", array)
n
and on
the size of the parameters Mean
, P
or
array
(see below for details).
The syntaxes
S = grand("getgen") grand("setgen", gen) S = grand("getsd") grand("setsd", S) grand("setcgn", G) S = grand("getcgn") grand("initgn", I) grand("setall", s1, s2, s3, s4) grand("advnst", K)
指定された分布から乱数を取得する
- ベータ分布
Y = grand(m,n, 'bet', A,B)
は パラメータA
およびdB
を指定したベータ分布からランダム変量を生成します. ベータ分布の密度は (0 < x < 1) です:A
およびB
は 実数で > 10-37とします. 関係する関数は : cdfbetです.- 二項分布
Y = grand(m,n, 'bin', N,p)
は パラメータN
(正の整数) およびp
([0,1]の範囲の実数)を指定した二項分布に基づきランダム変量を生成します. この分布では,成功する確率がp
のN
回の独立なベルヌーイ試行における 成功回数となります. 関係する関数: binomial, cdfbin.- 負の二項分布
Y = grand(m,n, 'nbn', N,p)
は パラメータN
(正の整数) およびp
( (0,1)の実数)を指定した負の二項分布に基づきランダム変量を生成します : 成功確率p
の独立なベルヌーイ試行においてN
回成功する前に発生する失敗の回数. 関係する関数 : cdfnbn.- カイ二乗分布
Y = grand(m,n, 'chi', Df)
は,自由度Df
(実数 > 0.0)を指定した カイ二乗分布に基づくランダム変量を生成します. 関係する関数: cdfchi.- 非心カイ二乗分布
Y = grand(m,n, 'nch', Df,Xnon)
は, 自由度Df
(実数 > 0.0)および 非心度パラメータXnonc
(実数 >= 0.0) を指定した非心カイ二乗分布に基づくランダム変量を生成します. 関係する関数 : cdfchn.- 指数分布
Y = grand(m,n, 'exp', Av)
は 平均Av
(実数 >= 0.0) を指定した指数分布に基づくランダム変量を生成します.- F分布
Y = grand(m,n, 'f', Dfn,Dfd)
は, 分子の自由度Dfn
(実数 > 0.0) および 分母の自由度Dfd
(実数 > 0.0) を指定した F(分散比)分布に基づくランダム変量を生成します. 関係する関数 : cdff.- 非心F分布
Y = grand(m,n, 'nf', Dfn,Dfd,Xnon)
は, 分子の自由度Dfn
(実数 >= 1), および 分母の自由度Dfd
(実数 > 0) , および非心パラメータXnonc
(実数 >= 0)を指定して 非心F分布に基づくランダム変量を生成します. 関係する関数 : cdffnc.- ガンマ分布
Y = grand(m,n, 'gam', shape,rate)
は,パラメータshape
(実数 > 0)およびrate
(実数 > 0)を指定して ガンマ分布に基づくランダム変量を生成します. ガンマの密度は以下となります :- ガウスラプラス(正規)分布
Y = grand(m,n, 'nor', Av,Sd)
は 平均Av
(実数) および標準偏差Sd
(実数 >= 0)の 正規分布に基づくランダム変量を生成します. 関係する関数 : cdfnor.- 多変量ガウス(多変量正規)分布
Y = grand(n, 'mn', Mean,Cov)
は,n
次の多変量正規ランダム変量を 生成します;Mean
はm x 1
行列,Cov
はm x m
対称正定行列です.Y
はこの場合m x n
行列となります.- 幾何分布
Y = grand(m,n, 'geom', p)
は, パラメータp
を指定した 幾何分布に基づくランダム変量を生成します: (成功率p
の)ベルヌーイ試行に1回成功するまでの回数.p
は [1.3e-307,1]の範囲とします.Y
は整数の値を有する正の実数となり, これらは "1回の成功を得る時の試行回数"です.- マルコフ分布
Y = grand(n, 'markov', P,x0)
は, 推移行列P
で記述されたマルコフ連鎖のn
個の一連の状態量を生成します. 状態量の初期値はx0
で指定されます.x0
が大きさm=size(x0,'*')
の 行列の場合,Y
は大きさm x n
の行列となります.Y(i,:)
は初期状態量x0(i)
から得られるサンプルパスです.- 多項分布
Y = grand(n, 'mul', nb,P)
は, 多項分布に基づきn
個の観測量を生成します:m
個のカテゴリの中にnb
個の分類のイベント (m
個の"boxes"の中にnb
個の"balls").P(i)
はあるイベントがカテゴリ i に分類される確率です. 確率ベクトルP
の大きさはm-1
です (カテゴリm
の確率は1-sum(P)
となります).Y
の大きさはm x n
で, 各列Y(:,j)
は多項分布の観測量,Y(i,j)
は(j
番目の観測値に関する)カテゴリi
に 分類されるイベントの数です(sum(Y(:,j)) = nb
).- ポワソン分布
Y = grand(m,n, 'poi', mu)
は, 平均mu (実数 >= 0.0)
のポワソン分布に基づき 乱数を生成します. 関係する機能 : cdfpoi.- ランダム置換
Y = grand(n, "prm", array)
generatesn
random permutations of thearray
elements.array
can be a vector, matrix or hypermatrix of real or complex numbers, of integers, booleans, polynomials or strings; full or sparse.- If
array
is a1 x m
row vector,Y
is an x m
matrix. - If
array
is am x 1
column vector,Y
is am x n
matrix. - If
array
is am x p
matrix,Y
is am x p x n
hypermatrix. - If
array
is am x p x q…
hypermatrix,Y
is am x p x q… x n
hypermatrix.
- If
- 一様分布 (def)
Y = grand(m,n, 'def')
は, は,[0,1)
(1は含まれません) の範囲の 一様分布に基づきランダム変量を生成します.- 一様分布 (unf)
Y = grand(m,n, 'unf', Low,High)
は[Low, High)
の範囲の一様分布に基づく の実数のランダム変量を生成します.- 一様分布 (uin)
Y = grand(m,n, 'uin', Low,High)
は,Low
およびHigh
(含む) の範囲の一様分布に基づく の整数のランダム変量を生成します.High
およびLow
は(High-Low+1) < 2147483561
の 範囲の整数とする必要があります.- 一様分布 (lgi)
Y = grand(m,n, 'lgi')
は カレントの生成器の基底出力として 以下の範囲の一様分布に基づくランダムな整数を返します:mt および kiss の場合は
[0, 2^32 - 1]
clcg2 の場合は
[0, 2147483561]
clcg4の場合は
[0, 2^31 - 2]
urandの場合は
[0, 2^31 - 1]
.
カレントの生成器およびその状態を設定/取得
Scilab-2.7以降,異なる('lgi'分布に基づくランダムな整数,もしくは その整数に基づき得られたもの)基底生成器を選択することが 可能となっています:
- mt
周期
2^19937
の M. Matsumoto および T. NishimuraのMersenne-Twister, 状態は624
個の整数の配列(およびこの配列のインデックス)により 指定されます; これがデフォルトの生成器です.- kiss
周期
2^123
のG. MarsagliaのKeep It Simple Stupid, 状態は4
個の整数により指定されます.- clcg2
周期
2^61
のP. L'Ecuyerの2線形共役合同生成器, 状態は2
個の整数により指定されます; grandにより以前に(若干修正された)使用されていた生成器です.- clcg4
周期
2^121
のP. L'Ecuyerの4線形合同生成器, 状態は4
個の整数により指定されます; この生成器は101
個の(重ならない)異なる生成器に 分割することができ,異なるタスクの場合に有用です ('clcg4に固有の動作'および 'clcg4のテスト例'を参照).- urand
scilab 関数 randで使用される生成器,
1
個の整数で状態が定義され, 周期は2^31
(d.e. knuth (1969), vol 2. State に示された理論と提案に基づく). これはこの一覧の中では高速ですが, やや古臭くなっています (重要なシミュレーションでは使用しないでください).
全ての生成器に共通なアクションの動作を以下に示します:
- action= 'getgen'
S = grand('getgen')
はカレントの基底生成器を返します (S
は以下の文字列のどれかです: 'mt', 'kiss', 'clcg2', 'clcg4', 'urand'.- action= 'setgen'
grand('setgen', gen)
は カレントの基底生成器をgen
に設定します. この文字列には 'mt', 'kiss', 'clcg2', 'clcg4', 'urand' のどれかを指定します( このコールは新しいカレントの生成器を返すことに注意してください).- action= 'getsd'
S = grand('getsd')
はカレントの基底生成器の カレントの状態 (カレントのシード)を返します ;S
には, mt の場合は625
次 (先頭は[1,624]
の範囲のインデックスです), kiss の場合は4
次, clcg2の場合は2
次, clcg4 の場合は4
次 (最後の1つについてはカレントの仮想生成器のカレントの状態が取得されます), および urand の場合は1
次 の(整数)列ベクトルが出力されます.- action= 'setsd'
grand('setsd', S), grand('setsd',s1[,s2,s3,s4])
は カレントの基底生成器の状態(新しいシード)を設定します:- mtの場合
S
は625
次の整数ベクトル (最初の要素はインデックスで[1,624]
の範囲とする 必要があります, 続く624
個の要素は[0,2^32[
)の範囲とします) (しかし,全てをゼロにするすることはできません); 整数s1
を1つだけ指定することで,より簡単に初期化を 行うことができます. (s1
は[0,2^32[
の範囲とします) ;- kissの場合
[0,2^32[
の範囲の4
個の整数s1,s2, s3,s4
を指定します ;- clcg2の場合
2
個の整数s1
(範囲:[1,2147483562]
) およびs2
(範囲:[1,2147483398]
) を指定します ;- clcg4の場合
4
個の整数s1
(範囲:[1,2147483646]
),s2
(範囲:[1,2147483542]
),s3
(範囲:[1,2147483422]
),s4
(範囲:[1,2147483322]
) を指定します ;注意
: clcg4の場合, カレントの仮想生成器のシードを設定しますが, この生成器と他の仮想生成器との間の同期が失われる可能性があります. すなわち,生成されるデータ列は他の生成器により生成されるデータ列と オーバーラップしていないということは保証されません) => 代わりに 'setall' オプションを使用してください.- urandの場合
[0,2^31
[の範囲の1
個の整数s1
を指定します.
- action= 'phr2sd'
Sd = grand('phr2sd', phrase)
は, 基底生成器(clcg2に適しています)の状態を変更するシードとして使用可能な1 x 2
ベクトルSd
を生成するphrase
(文字列)を指定します.
clcg4の固有のオプション
clcg4 生成器は他の生成器と同時に使用することができますが,
系列がオーバーラップしない
複数(101
個)の仮想生成器に分割できるという利点があります.
(古典的な生成器を使用する場合,異なる系列を得るために
初期状態量(シード)を別のものに変更できますが,完全に異なる系列となることは
保証されません)
各仮想生成器は2^72
個の値の系列に対応します.
これらはさらに長さW=2^41
のV=2^31
個の
セグメント(またはブロック)に分割されます.
指定した仮想生成器について,系列の先頭またはカレントのセグメントの先頭に戻るか
次のセグメントに直接移動するかを選択できます.
'setall'オプションにより生成器 0
の初期状態(シード)を
変更することも可能です.
これにより,同期をとるために他の仮想生成器の初期状態量を変更することも可能です.
(すなわち, gen 0
の新しい初期状態の関数として,
gen 1..100
の初期状態量は101
個の
重複しない系列が得られるように再計算されます.)
- action= 'setcgn'
grand('setcgn', G)
は clcg4 のカレントの仮想生成器を設定します (clcg4 が設定された時, この値は使用される仮想 (clcg4)生成器の数G
となります); 仮想 clcg4 生成器は0,1,..,100
と番号付けられます (そして,G
は[0,100]
の範囲の整数となります); デフォルトで,カレントの仮想生成器は0
です.- action= 'getcgn'
S = grand('getcgn')
はカレントの仮想 clcg4 生成器の数を返します.- action= 'initgn'
grand('initgn', I)
はカレントの仮想生成器の状態を再度初期化します- I = -1
初期シードの状態を設定します
- I = 0
直近の(前の)シードを設定します (つまり,カレントのセグメントの先頭)
- I = 1
直近のシード(すなわち,次のセグメントの先頭)から新しいシード
W
に 状態量を設定し,カレントのセグメントのパラメータをリセットします.
- action= 'setall'
grand('setall',s1,s2,s3,s4)
は 生成器0
の初期状態をs1,s2,s3,s4
に設定します. 他の生成器の初期シードは同期がとれるように設定されます.s1, s2, s3, s4
に関する制約については,'setsd'アクションを参照ください.- action= 'advnst'
grand('advnst',K)
はカレントの生成器の状態を2^K
だけ前に進め,初期シードをその値でリセットします.
例
In the following example, we generate random numbers from various distributions and plot the associated histograms.
// Returns a 400 x 800 matrix of random doubles, // with normal distribution and average 0 and standard deviation 1. R = grand(400,800,"nor",0,1); scf(); histplot(10,R); xtitle("Normal random numbers from grand","X","Frequency");
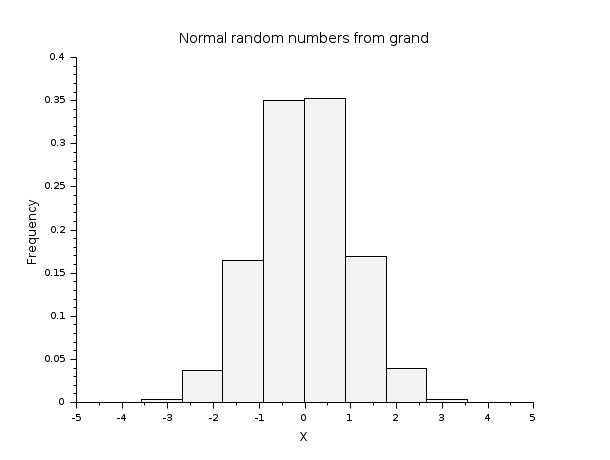
// Returns a 400 x 800 matrix of random doubles, // uniform in [0,1). R = grand(400,800,"def"); scf(); histplot(10,R); xtitle("Uniform random numbers from grand","X","Frequency");
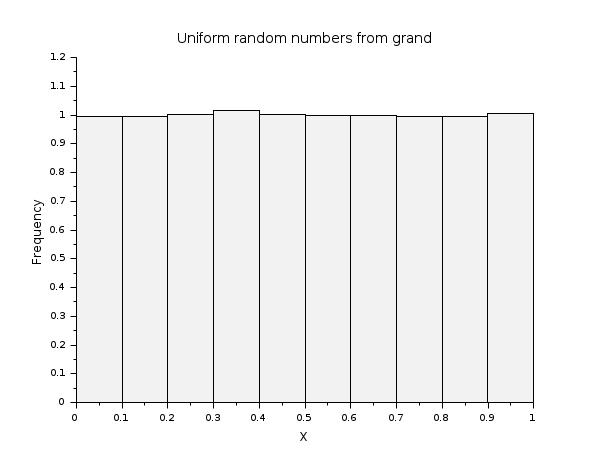
// Returns a 400 x 800 matrix of random doubles, // with Poisson distribution and average equal to 5. R = grand(400,800,"poi",5); scf(); histplot(10,R); xtitle("Poisson random numbers from grand","X","Frequency");
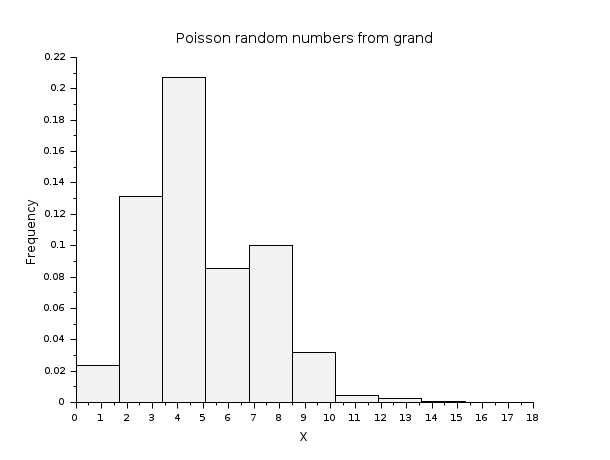
In the following example, we generate random numbers from the exponential distribution and then compare the empirical with the theoretical distribution.
lambda=1.6; N=100000; X = grand(1,N,"exp",lambda); scf(); classes = linspace(0,12,25); histplot(classes,X); x=linspace(0,12,25); y = (1/lambda)*exp(-(1/lambda)*x); plot(x,y,"ro-"); legend(["Empirical" "Theory"]); xtitle("Exponential random numbers from grand","X","Frequency");
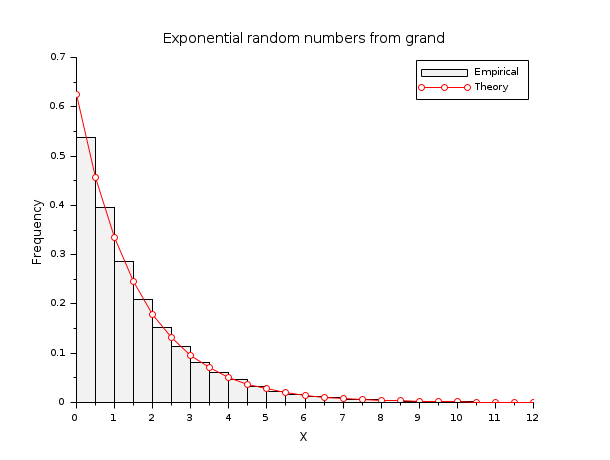
In the following example, we generate random numbers from the gamma distribution and then compare the empirical with the theoretical distribution.
N=10000; A=10; B=4; R=grand(1,N,"gam",A,B); XS=gsort(R,"g","i")'; PS=(1:N)'/N; P=cdfgam("PQ",XS,A*ones(XS),B*ones(XS)); scf(); plot(XS,PS,"b-"); // Empirical distribution plot(XS,P,"r-"); // Theoretical distribution legend(["Empirical" "Theory"]); xtitle("Cumulative distribution function of Gamma random numbers","X","F");
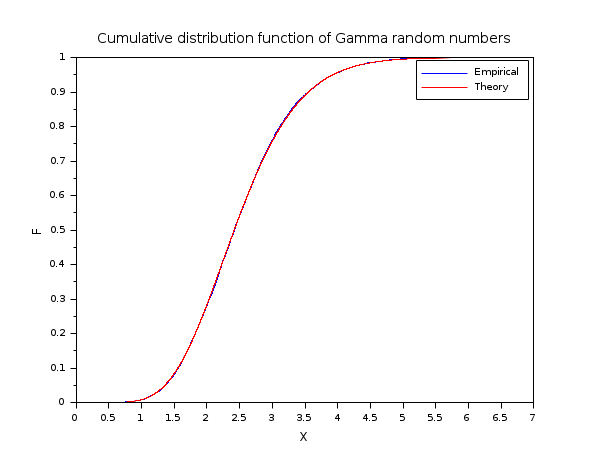
In the following example, we generate 10 random integers in the [1,365] interval.
grand(10,1,"uin",1,365)
In the following example, we generate 12 permutations of the [1,2,...,7] set. The 12 permutations are stored column by column.
grand(12,"prm",(1:7)')
In the following example, we generate a
10
×10
×10
hypermatrix of random numbers from the "normal" distribution and plot the associated histograms.
Showing the first and last layers.
// Returns a 10 x 10 x 10 hypermatrix of random doubles, // with normal distribution, average 0 and standard deviation 1. // Showing the first and last layers. R = grand(10,10,10,"nor",0,1); subplot(1,2,1) bar3d(R(:,:,1)); xtitle("Layer 1"); subplot(1,2,2) bar3d(R(:,:,10)); xtitle("Layer 10");
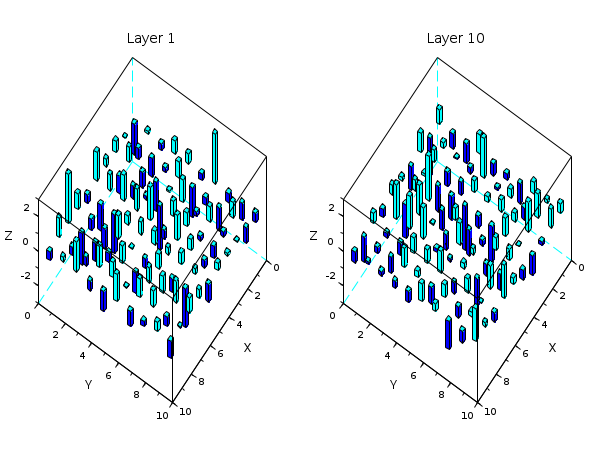
Get predictable or less predictable numbers
The pseudo random number generators are based on deterministic sequences.
In order to get reproducible simulations, the initial seed of the generator is constant,
such that the sequence will remain the same from a session to the other.
Hence, by default, the first numbers produced by grand
are always
the same.
In some situations, we may want to initialize the seed of the generator in
order to produce less predictable numbers.
In this case, we may initialize the seed with the output of the getdate
function:
n = getdate("s"); grand("setsd",n)
clcg4のテスト例
clcg4 の分割機能の適用例を以下に示します. 2つの統計上のテクニックを異なるデータの大きさで比較します. 最初の手法では,ブートストラップ手法を使用し,しらみつぶし法のみを適用する 2番目の手法よりも少ないデータで同等の精度が得られると考えられます. 最初の方法の場合,25および50の間に一様分布する大きさのデータ集合が生成されます. その後,指定した大きさのデータ集合が生成され,解析されます. 2番目の方法は, 100および200の間の大きさのデータ集合を選択し,データを生成し,解析します. この過程を1000回繰り返します. 分散を減少させるために,2つの手法で使用される乱数を1000回の比較の各回で 同じとすることが望まれます. しかし,2番目の手法は1番目の手法よりも多くの乱数を使用し, このパッケージなしでは同期は困難となります. clcg4 ではこれは容易です. 生成器 0 を使用し最初の手法のサンプルの大きさを取得し, 生成器 1 をデータを得る際に使用します. 次にカレントのブロックの先頭に状態をリセットし, 2番目の手法にも同様のことをします. これにより,2番目の手法で使用される初期データが1番目の手法で使用されることが 保証されます. 両方の処理が完了した時,両方の生成器のブロックを前に進めます.
参照
- rand — 乱数生成
- sprand — ランダム疎行列
- ssrand — 乱数生成器
- randpencil — ランダムなペンシル
- genmarkov — 再帰的および一時的なクラスを有するランダムなマルコフ行列を生成する
Report an issue | ||
<< random | random | prbs_a >> |