Please note that the recommended version of Scilab is 2025.0.0. This page might be outdated.
See the recommended documentation of this function
lqe
linear quadratic estimator (Kalman Filter)
Syntax
[K,X]=lqe(Pw)
[K,X]=lqe(P,Qww,Rvv [,Swv])
Arguments
- Pw
A state space representation of a linear dynamical system (see syslin)
- P
A state space representation of a linear dynamical system (
nu
inputs,ny
outputs,nx
states) (see syslin)- Qww
Real
nx
bynx
symmetric matrix, the process noise variance- Rvv
full rank real
ny
byny
symmetric matrix, the measurement noise variance.- Swv
real
nx
byny
matrix, the process noise vs measurement noise covariance. The default value is zeros(nx,ny).- K
a real matrix, the optimal gain.
- X
a real symmetric matrix, the stabilizing solution of the Riccati equation.
Description
This function computes the linear optimal LQ estimator gain of the state estimator for a detectable (see dt_ility) linear dynamical system and the variance matrices for the process and the measurement noises.
Syntax [K,X]=lqe(P,Qww,Rvv [,Swv])
Computes the linear optimal LQ estimator gain K for the dynamical system:


Where are white noises such
as
![]() | CautionThe values of |
![]() | Standard form![]() ![]() ![]() ![]() ![]() ![]() |
Syntax [K,X]=lqe(Pw)
Computes the linear optimal LQ estimator gain K for the dynamical system

Where is a white noise with unit covariance.
Properties
is stable.
the state estimator is given by the dynamical system:
or
It minimizes the covariance of the steady state error.
For discrete time systems the state estimator is such that:
(one-step predicted
).
Algorithm
let ,
and
For the continuous time case K is given by
where
X
is the solution of the stabilizing Riccati equationFor the discrete time case K is given by
where
X
is the solution of the stabilizing Riccati equation
Examples
Assume the dynamical system formed by two masses connected by a spring and a damper:
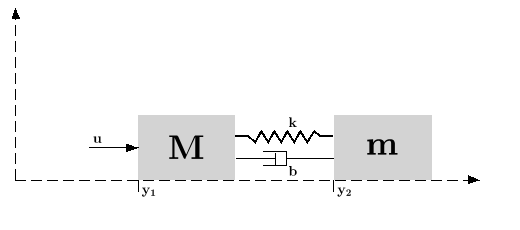





A continuous time state space representation of this system is:
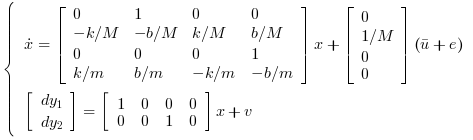
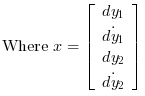



The instructions below can be used to compute a LQ state observer of the discretized version of this dynamical system.
// Form the state space model M = 1; m = 0.2; k = 0.1; b = 0.004; A = [ 0 1 0 0 -k/M -b/M k/M b/M 0 0 0 1 k/m b/m -k/m -b/m]; B = [0; 1/M; 0; 0]; C = [1 0 0 0 //dy1 0 0 1 0];//dy2 //inputs u and e; outputs dy1 and dy2 P = syslin("c",A, B, C); // Discretize it dt=0.5; Pd=dscr(P, dt); // Set the noise covariance matrices Q_e=0.01; //additive input noise variance R_vv=0.001*eye(2,2); //measurement noise variance Q_ww=Pd.B*Q_e*Pd.B'; //input noise adds to regular input u //----syntax [K,X]=lqe(P,Qww,Rvv [,Swv])--- Ko=lqe(Pd,Q_ww,R_vv); //observer gain //----syntax [K,X]=lqe(P21)--- bigR =sysdiag(Q_ww, R_vv); [W,Wt]=fullrf(bigR); Bw=W(1:size(Pd.B,1),:); Dw=W(($+1-size(Pd.C,1)):$,:); Pw=syslin(Pd.dt,Pd.A,Bw,Pd.C,Dw); Ko1=lqe(Pw); //same observer gain //Check gains equality norm(Ko-Ko1,1) // Form the observer O=observer(Pd,Ko); //check stability and(abs(spec(O.A))<1) // Check by simulation // Modify Pd to make it return the state Pdx=Pd;Pdx.C=eye(4,4);Pdx.D=zeros(4,1); t=0:dt:30; u=zeros(t); x=flts(u,Pdx,[1;0;0;0]);//state evolution y=Pd.C*x; // simulate the observer x_hat=flts([u+0.01*rand(u);y+0.0001*rand(y)],O); clf; subplot(2,2,1) plot2d(t',[x(1,:);x_hat(1,:)]'), e=gce();e.children.polyline_style=2; L=legend('$x_1=dy_1$', '$\hat{x_1}$');L.font_size=3; xlabel('Time (s)') subplot(2,2,2) plot2d(t',[x(2,:);x_hat(2,:)]') e=gce();e.children.polyline_style=2; L=legend('$x_2=dy_1^+$', '$\hat{x_2}$');L.font_size=3; xlabel('Time (s)') subplot(2,2,3) plot2d(t',[x(3,:);x_hat(3,:)]') e=gce();e.children.polyline_style=2; L=legend('$x_3=dy_2$', '$\hat{x_3}$');L.font_size=3; xlabel('Time (s)') subplot(2,2,4) plot2d(t',[x(4,:);x_hat(4,:)]') e=gce();e.children.polyline_style=2; L=legend('$x_4=dy_2^+$', '$\hat{x_4}$');L.font_size=3; xlabel('Time (s)')
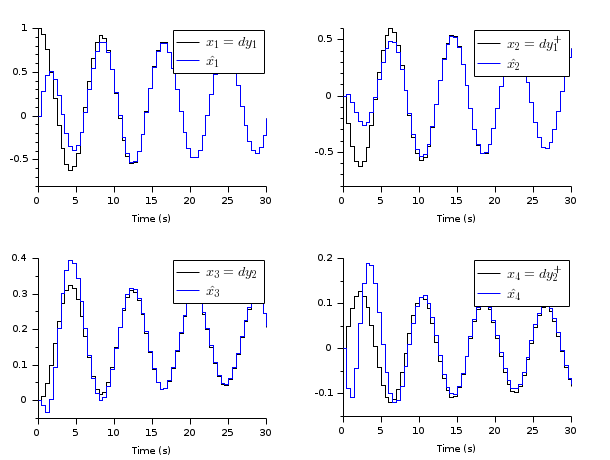
Reference
Engineering and Scientific Computing with Scilab, Claude Gomez and al.,Springer Science+Business Media, LLC,1999, ISNB:978-1-4612-7204-5
See also
Report an issue | ||
<< leqr | Linéaire Quadratique | lqg >> |