dsearch
distribute, locate and count elements of a matrix or hypermatrix in given categories
Syntax
[i_bin [,counts [,outside]]] = dsearch(X, bins ) [i_bin [,counts [,outside]]] = dsearch(X, bins , pm )
Arguments
- X
matrix or hypermatrix of reals, encoded integers, or texts: The entries to categorize. Complex numbers and polynomials are not supported.
- bins
row or column vector defining categories, of same type as
X
(for encoded integers inX
,bins
may be decimals).- Discrete case (pm="d"):
bins
are distinct values to whichX
entries must be identified. IfX
is numeric (reals or encoded integers),bins
must be sorted in strictly increasing order. - Continuous case (default, pm="c"):
bins
are bounds of contiguous intervals:I1 = [bins(1), bins(2)]
,I2 = (bins(2), bins(3)]
,...,In = (bins(n), bins(n+1)]
. Note that entries fromX
just equal to bins(1) are included inI1
. The values inbins
must be in strictly increasing order: bins(1) < bins(2) < ... < bins(n). For text processing, the case-sensitive lexicographic order is considered.
- Discrete case (pm="d"):
- pm
"c" (continuous, default) or "d" (discrete): processing mode. In continuous mode,
bins
defines the bounds of contiguous intervals considered as categories. In discrete mode,bins
provides the values to which entries fromX
must be identified.- i_bin
matrix or hypermatrix with same sizes than
X
:i_bin(k)
is the index of the category to whichX(k)
belongs. IfX(k)
belongs to none of the categories,i_bin(k) = 0
- counts
- number of X entries in respective bins.
Continuous case (pm="c"): counts(i) elements of
X
belong to the intervalIk
as defined above (see thebins
parameter). Elements ofX
just equal to bins(1) are included in counts(1).counts
has the size ofbins
- 1Discrete case (pm="d"):
counts(i)
elements ofX
are equal tobins(i)
- outside
Total number of X entries belonging to none of the
bins
.
Description
For each X(i)
entry, dsearch
locates the value bins(j)
or the interval (bins(j), bins(j+1)]
defined by bins
and containing or equal to X(i)
. Then it returns i_bin(i) = j
or 0 whether no bin equals or contains it. (the first interval includes bins(1)
). The population of each bin is returned through counts
. The total number of unbinned entries is returned in outside
(therefore outside = sum(bool2s(i_bin==0))
).
dsearch(..)
can be overloaded.
The default pm="c" option can be used to compute the empirical histogram of a function given a dataset.
Examples
// DISCRETE values of TEXT // ----------------------- i = grand(4,6,"uin",0,7)+97; T = matrix(strsplit(ascii(i),1:length(i)-1), size(i)); T(T=="f") = "#"; T(T=="a") = "AA"; T bins = [ strsplit(ascii(97+(7:-1:0)),1:7)' "AA"] [i_bin, counts, outside] = dsearch(T, bins, "d") // BINNING TEXTS in LEXICOGRAPHIC INTERVALS // ---------------------------------------- // generating a random matrix of text nL = 3; nC = 5; L = 3; s = ascii(grand(1,nL*nC*L,"uin",0,25)+97); T = matrix(strsplit(s, L:L:nL*nC*L-1), nL, nC); // generating random bins bounds L = 2; nC = 6; s = ascii(grand(1,nC*L,"uin",0,25)+97); bins = unique(matrix(strsplit(s, L:L:nC*L-1), 1, nC)) T [i_bin, counts, outside] = dsearch(T, bins)
In the following example, we consider 3 intervals I1 = [5,11]
,
I2 = (11,15]
and I3 = (15,20]
.
We are looking for the location of the entries of X = [11 13 1 7 5 2 9]
in these intervals.
[i_bin, counts, outside] = dsearch([11 13 1 7 5 2 9], [5 11 15 20])
Displayed output:
-->[i_bin, counts, outside] = dsearch([11 13 1 7 5 2 9], [5 11 15 20]) outside = 2. counts = 4. 1. 0. i_bin = 1. 2. 0. 1. 1. 0. 1.
Indeed,
X(1)=11 is in the interval I1, hence i_bin(1)=1.
X(2)=13 is in the interval I2, hence i_bin(2)=2.
X(3)=1 belongs to none of defined intervals, hence i_bin(3)=0.
X(4)=7 is in the interval I1, hence i_bin(4)=1.
...
There are four X entries (5, 7, 9 and 11) in I1, hence counts(1)=4.
There is only one X entry (13) in I2, hence counts(2)=1.
There is no X entry in I3, hence counts(3)=0.
There are two X entries (i.e. 1, 2) which belong to none of defined intervals, hence outside=2.
// Numbers in DISCRETE categories (having specific values) // ------------------------------ [i_bin, counts, outside] = dsearch([11 13 1 7 5 2 9], [5 11 15 20],"d" )
displays
-->[i_bin, counts, outside] = dsearch([11 13 1 7 5 2 9], [5 11 15 20], "d" ) outside = 5. counts = 1. 1. 0. 0. i_bin = 2. 0. 0. 0. 1. 0. 0.
Indeed,
X(1)=11 is in the set
bins
at position #2, hence i_bin(1)=2.X(2)=13 is not in the set
bins
, hence i_bin(2)=0....
X(7)=9 is not in the set
bins
, hence i_bin(7)=0.There is only one entry X (i.e. 5) equal to 5, hence counts(1)=1.
There are no entries matching
bins(4)
, hence counts(4)=0.There are five X entries (i.e. 13, 1, 7, 2, 9) which are not in the set
bins
, hence outside=5.
Numbers in bins
must be in increasing order, whatever is the processing mode (continuous or discrete).
If this is not the case, an error is generated:
-->dsearch([11 13 1 7 5 2 9], [2 1]) !--error 999 dsearch : the array s (arg 2) is not well ordered -->dsearch([11 13 1 7 5 2 9], [2 1],"d") !--error 999 dsearch : the array s (arg 2) is not well ordered
Advanced Examples
In the following example, we compare the empirical histogram of uniform random numbers in [0,1) with the uniform distribution function. To perform this comparison, we use the default search algorithm based on intervals (pm="c"). We generate X as a collection of m=50 000 uniform random numbers in the range [0,1). We consider the n=10 values equally equally spaced values in the [0,1] range and consider the associated intervals. Then we count the number of entries in X which fall in the intervals: this is the empirical histogram of the uniform distribution function. The expectation for counts/m is equal to 1/(n-1).
m = 50000 ; n = 10; X = grand(m, 1, "def"); bins = linspace(0, 1, n)'; [i_bin, counts] = dsearch(X, bins); e = 1/(n-1)*ones(1, n-1); scf() ; plot(bins(1:n-1), counts/m, "bo"); plot(bins(1:n-1), e', "r-"); legend(["Experiment", "Expectation"]); xtitle("Uniform random numbers", "X", "P(X)");
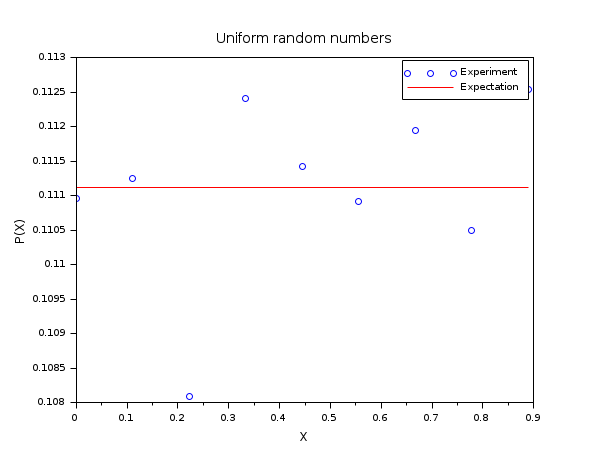
In the following example, we compare the histogram of binomially distributed random numbers with the binomial probability distribution function B(N,p), with N=8 and p=0.5. To perform this comparison, we use the discrete search algorithm based on a set (pm="d").
N = 8 ; p = 0.5; m = 50000; X = grand(m,1,"bin",N,p); bins = (0:N)'; [i_bin, counts] = dsearch(X, bins, "d"); Pexp = counts/m; Pexa = binomial(p,N); scf() ; plot(bins, Pexp, "bo"); plot(bins, Pexa', "r-"); xtitle("Binomial distribution B(8,0.5)","X","P(X)"); legend(["Experiment","Expectation"]);
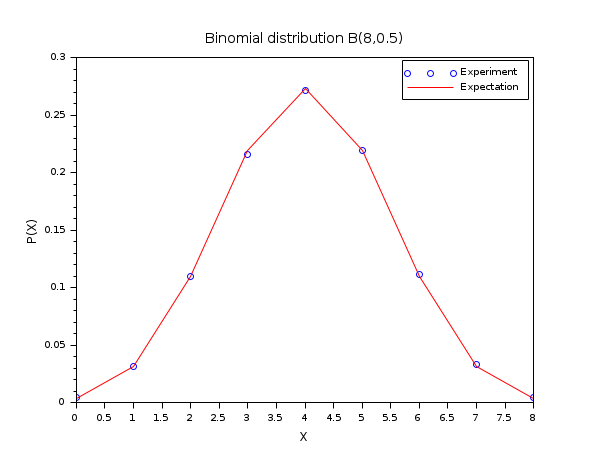
In the following example, we use piecewise Hermite polynomials to interpolate a dataset.
// define Hermite base functions function y=Ll(t, k, x) // Lagrange left on Ik y=(t-x(k+1))./(x(k)-x(k+1)) endfunction function y=Lr(t, k, x) // Lagrange right on Ik y=(t-x(k))./(x(k+1)-x(k)) endfunction function y=Hl(t, k, x) y=(1-2*(t-x(k))./(x(k)-x(k+1))).*Ll(t,k,x).^2 endfunction function y=Hr(t, k, x) y=(1-2*(t-x(k+1))./(x(k+1)-x(k))).*Lr(t,k,x).^2 endfunction function y=Kl(t, k, x) y=(t-x(k)).*Ll(t,k,x).^2 endfunction function y=Kr(t, k, x) y=(t-x(k+1)).*Lr(t,k,x).^2 endfunction x = [0 ; 0.2 ; 0.35 ; 0.5 ; 0.65 ; 0.8 ; 1]; y = [0 ; 0.1 ;-0.1 ; 0 ; 0.4 ;-0.1 ; 0]; d = [1 ; 0 ; 0 ; 1 ; 0 ; 0 ; -1]; X = linspace(0, 1, 200)'; i_bin = dsearch(X, x); // plot the curve Y = y(i_bin).*Hl(X,i_bin) + y(i_bin+1).*Hr(X,i_bin) + d(i_bin).*Kl(X,i_bin) + d(i_bin+1).*Kr(X,i_bin); scf(); plot(X,Y,"k-"); plot(x,y,"bo") xtitle("Hermite piecewise polynomial"); legend(["Polynomial","Data"]); // NOTE : it can be verified by adding these ones : // YY = interp(X,x,y,d); plot2d(X,YY,3,"000")
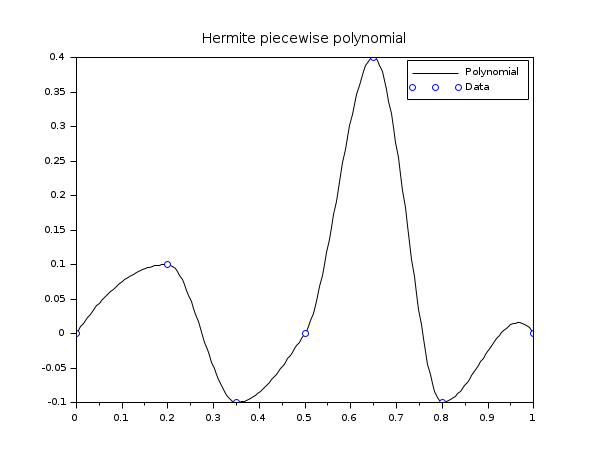
See also
History
Version | Description |
5.5.0 | Extension to hypermatrices, encoded integers, and text. |
Report an issue | ||
<< Search and sort | Search and sort | find >> |